Governments Should Independently Audit AI Tools For Fairness: Analytics Expert
This article originally appeared on Which-50
Data and analytics have enormous potential to improve public policy and services by helping governments focus their resources in the areas where they will be most effective. However the risk of deploying machine learning systems which unfairly impact humans lives, because they’ve inherited biases from their human designers, means a new market may emerge for tools and services to audit algorithms.
Rayid Ghani Director, Center for Data Science and Public Policy University of Chicago, argues that machine learning systems should be audited by a third party and the results made public before any models are widely deployed by government departments.
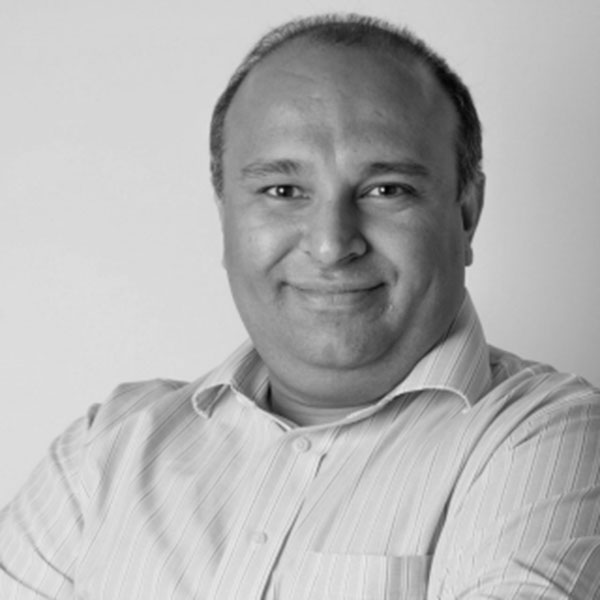
Rayid Ghani
Director, Center for Data Science and Public Policy
University of Chicago
Research Expertise: Analytics, Data Mining, Machine Learning, Social Media Analytics, Text Analytics, Natural Language Processing, Social Networks.
Recently (and reluctantly) added buzzwords: Big Data, Data Science, Artificial Intelligence
Older buzzwords that are trendy now: Machine Learning
Not so old buzzwords that are not trendy now: Data Mining
I’m interested in using computation, data and analytics for solving high impact social good problems in areas such as criminal justice, education, healthcare, energy, transportation, economic development, and public safety.
I am the Director of the Center for Data Science and Public Policy, Research Director and Senior Fellow at the Computation Institute and a Senior Fellow at the Harris School of Public Policy at the University of Chicago.
What I used to do: Chief Scientist at Obama for America 2012 campaign focusing on analytics, technology, and data. Senior Research Scientist and Director of Analytics research at Accenture Labs where I led a technology research team focused on applied R&D in analytics, machine learning, and data mining for large-scale & emerging business problems in various industries including healthcare, retail & CPG, manufacturing, intelligence, and financial services.
In my ample free time, I advise several analytics start-ups and non-profits, speak at, organize and participate in academic and industry analytics conferences, and publish in machine learning and data mining conferences and journals.
An advocate for the way data can be used to improve society, Ghani works with governments and non-profits to help them conduct analytics projects with a focus on developing policies to create a more equitable society.
“There’s a lot of good things we can do with data but we need to make sure we train people to think about these other concerns and build tools to make it easier for people to increase equity when doing analytics, machine learning and AI,” Ghani told Which-50.
Spurred on by advances in the private sector, Ghani says governments are now realising the value of data but cautioned they must adopt ethical approaches to reduce the risk of bias.
The dangers of algorithmic bias were exemplified last week, when Reuters revealed Amazon built (and later scrapped) a recruitment algorithm which was biased against women. The model, trained mostly on men’s resumes, penalising any application that contained the word “women’s”, such as women’s sports teams or colleges.
Awareness of the ethical issues surrounding artificial intelligence is rising. According to data from CB Insights, news mentions of AI and ethics increased almost 5000 per cent from 2014 to 2018, when they reached over 250 mentions in Q3 2018.
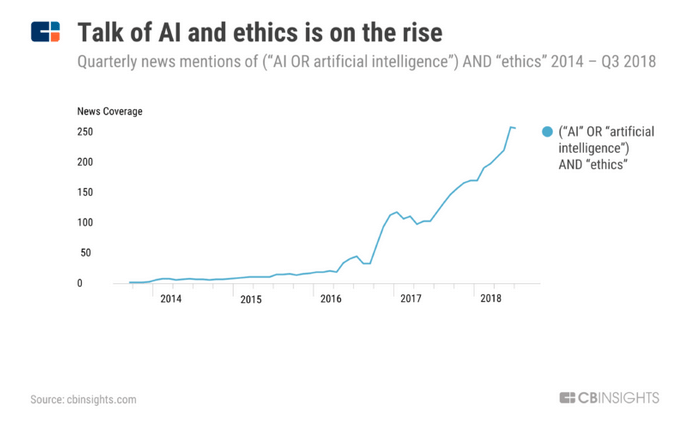
Driving the conversation is the concern is that is if it isn’t clear how models come up with their predictions or recommendations, then unfair decisions may be automated and go unchecked.
Countering Bias
According to Ghani, the first step to tackling the risk is to clearly define “where does fairness come in” to the problem you are using data to solve.
For example, Ghani has worked with the the public health department of Chicago to build a machine learning model to predict which children may be exposed to lead paint in their homes.
Without the resources to proactively fix every home with lead paint, the city has turned to a machine learning system to prioritise which homes to fix first based on 15 years worth of blood tests from children and home inspection data.
Ghani explained the goal to reduce the overall rate of lead poisoning needs to be framed in a manner that takes fairness into account. For example ‘how do I make sure the rate of lead poisoning for people living in one part of the city is as close to the rate for people living in the other parts of the city?’
“So I want to reduce [lead poisoning] overall, but I want to make sure that it is not being reduced disportionately for richer people or more educated people because I want to reduce the disparity,” Ghani explained.
“You want to put the metrics in place so you can measure the ability not just to execute the project but also to achieve these goals.”
AI Auditors
Once an analytics program has clearly defined goals which take fairness and equity into account, a third party (ie not the developer) should audit the system to measure how it is performing against those metrics, Ghani argues.
“The results of those audits should be made public before you can go and implement such a system,” Ghani said.
Ghani developed an open-source software called Aequitas to help audit machine learning tools for bias. He explained to Which-50 that the tool doesn’t fix the problem, but it lets you know when you’ve got one.
“The tool that we built was really a way to show people that when we are using predictive tools of any sort, they are going to make mistakes and those mistakes need to be thought about very carefully – certain types of mistakes are more costly than others.”
Aequitas examines the predictions a system has made to see if certain groups are scoring higher levels of false positives or false negatives.
Ghani argues this practice of defining goals and publicly auditing algorithms should become a part of compliance processes as data is used more widely to tackle policy issues.